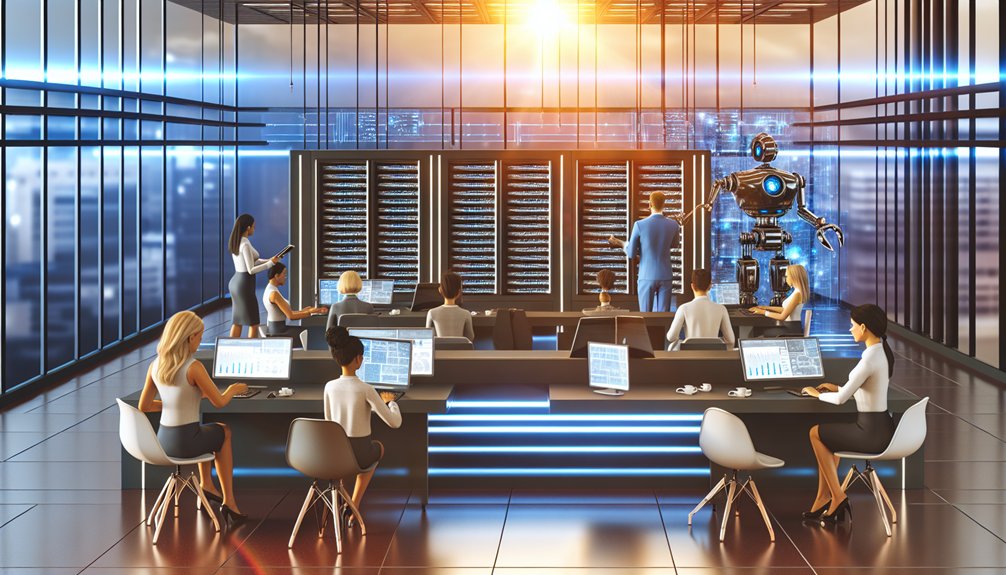
In AI journalism, machine learning is essential for efficiency and innovation. Automate data collection and cleaning to guarantee precision. Utilize NLP for summarizing articles and revealing trends. Sentiment analysis gauges audience emotions, crafting engaging content. Machine-driven storytelling boosts speed and accuracy, while optimization guarantees relevance. Keep in mind ethical considerations like bias and transparency. If you want to stay at the forefront of AI journalism advancements, you’ll find these techniques indispensable.
Data Collection and Preprocessing for AI Journalism
In AI journalism, data collection and preprocessing are essential for guaranteeing accurate and insightful content generation. You begin with data sourcing, identifying reputable sources that offer reliable and diverse datasets. Automation tools streamline this process, letting you gather vast amounts of information efficiently. Once collected, focus on data cleaning. This involves removing duplicates, correcting errors, and dealing with missing values. Clean data guarantees your AI models produce precise insights, reducing the risk of inaccuracies. By maintaining data quality, you empower AI systems to generate content that’s not only relevant but also trustworthy. As you master these steps, you’ll enhance your AI-driven journalism, delivering stories that resonate with clarity and precision. Remember, the foundation of compelling AI journalism lies in meticulous data handling.
Natural Language Processing Techniques in Newsrooms
While exploring the transformative role of Natural Language Processing (NLP) in newsrooms, you’ll discover its capacity to revolutionize content creation and analysis. Automated summarization tools can efficiently condense lengthy articles, ensuring your audience gains key insights quickly without sifting through endless text. This streamlining of information not only saves time but enhances reader engagement. Additionally, topic modeling uncovers underlying themes within vast datasets, helping you identify emerging trends or niche interests. By applying these techniques, you’re equipped to produce data-driven articles that resonate with your audience’s interests. As the media landscape evolves, leveraging NLP’s capabilities in automated summarization and topic modeling will position you at the forefront of innovative journalism, making your newsroom a hub of insightful, timely content.
Sentiment Analysis for News Content
You can automatically assess the emotional tone of news articles by applying sentiment analysis, giving you valuable insights into how content might affect readers. By leveraging algorithms, you can effectively analyze public reactions and identify sentiment trends, allowing for data-driven editorial decisions. This approach not only enhances understanding of audience engagement but also helps in curating content that resonates with readers.
Understanding Emotional Tone
Decoding the emotional tone of news content, sentiment analysis leverages machine learning to provide insights into how a story might impact its audience. By employing algorithms that excel in tone detection, you can gauge the emotional undertones of articles, enhancing your emotional intelligence as a journalist. Machine learning tools analyze textual data, identifying whether the tone is positive, negative, or neutral. This analysis aids in understanding the emotional weight of words and phrases, allowing you to craft content with greater awareness of its potential impact. Automated processes streamline this analysis, offering real-time insights into how your audience might feel. With these data-driven techniques, you can fine-tune your content strategy, ensuring it resonates emotionally and effectively with readers.
Analyzing Public Reactions
Understanding how the public reacts to news content is essential in shaping effective journalism. By leveraging sentiment analysis, you can automatically gauge public sentiment toward your articles. This technique involves analyzing text data from comments, social media, and forums to determine whether public sentiment is positive, negative, or neutral. It’s a powerful tool that allows you to tailor content to enhance audience engagement.
Automated sentiment analysis tools offer deep insights by processing vast amounts of data quickly, helping you identify which topics resonate most with your audience. Understanding these reactions can guide you in creating content that meets readers’ expectations and increases their engagement. By consistently analyzing public sentiment, you guarantee your journalism remains relevant and impactful in a rapidly changing media landscape.
Identifying Sentiment Trends
Identifying sentiment trends in news content plays a pivotal role in shaping stories that engage and resonate with readers. By analyzing sentiment patterns, you can uncover the emotions driving public discourse. With automated tools, you’ll efficiently track these patterns across vast datasets, providing real-time insights into audience mood. This understanding allows for precise trend forecasting, enabling you to predict shifts in public opinion and tailor content accordingly. Data-driven sentiment analysis offers a deeper connection with your audience, fostering stories that not only inform but also engage emotionally. It’s essential to integrate these insights into your editorial strategy, ensuring your news content remains relevant and impactful in an ever-evolving media landscape. Leverage these techniques for a competitive edge.
Automated Content Generation With Machine Learning
When harnessed effectively, automated content generation with machine learning revolutionizes how information is produced and disseminated in journalism. You’ll find that automated storytelling tools analyze vast datasets in real-time, crafting coherent narratives without human intervention. This technology not only speeds up content creation but also enhances accuracy by minimizing human error. With content optimization, machine learning algorithms refine your stories to engage audiences better, ensuring relevance and timeliness.
Personalization Algorithms for News Delivery
In the rapidly evolving landscape of digital journalism, personalization algorithms play an essential role in tailoring news delivery to individual preferences. By leveraging user profiling and audience segmentation, you can deliver content recommendations that boost user engagement. These algorithms utilize preference learning to understand what resonates with you by analyzing your interactions. Feedback loops are vital, as they allow the system to refine content suggestions based on your responses, optimizing the algorithm over time. Through relevance ranking, articles are prioritized based on their predicted value to you, guaranteeing that the most pertinent information reaches you first. Algorithm optimization guarantees that the system continuously adapts, providing a seamless experience that keeps you informed and engaged with the news that matters most to you.
Ethical Considerations in AI-Driven Journalism
When you implement machine learning in journalism, it’s essential to recognize how bias can infiltrate algorithm design, potentially skewing news delivery. Automated systems must guarantee data privacy and ethical data use to maintain public trust. By focusing on these ethical considerations, you can enhance the integrity and reliability of AI-driven journalism.
Bias in Algorithm Design
Although artificial intelligence has revolutionized journalism by streamlining content creation and distribution, bias in algorithm design presents significant ethical challenges. You must focus on algorithmic accountability and fairness metrics to guarantee that your AI tools promote unbiased reporting. Implement bias mitigation strategies and transparency measures to identify and correct skewed outcomes. Rely on diverse datasets and inclusive design to prevent homogeneous perspectives from influencing your reports. Regularly seek user feedback for ongoing improvement and perform impact assessments to understand the consequences of your AI’s decisions. Utilize ethical frameworks and thorough model evaluation to align your algorithms with journalistic standards. By prioritizing these aspects, you’ll create AI-driven content that upholds fairness and accuracy, maintaining public trust in journalism.
Privacy and Data Use
As AI revolutionizes journalism, safeguarding privacy and data use becomes essential for maintaining ethical standards. You need to focus on implementing effective data anonymization techniques to protect individual identities while gathering insights. Ensuring user consent policies are transparent and robust helps build trust and compliance with regulations.
Consider these key strategies:
- Data Anonymization Techniques: Use methods like pseudonymization and data masking to prevent identification.
- User Consent Policies: Clearly outline data usage terms and obtain explicit consent before data collection.
- Audit Trails: Maintain records of consent and data access to enhance accountability.
- Limit Data Collection: Gather only necessary data to minimize risks.
- Regular Reviews: Continuously assess and update privacy practices to stay aligned with evolving standards.
These steps help you maintain ethical AI journalism.
Frequently Asked Questions
How Can Machine Learning Improve the Accuracy of News Forecasting?
You can enhance news prediction accuracy by leveraging machine learning for data analysis. It automates pattern recognition, identifies trends, and provides insightful forecasts. This helps you make informed decisions and delivers a more precise understanding of future events.
What Are the Challenges of Integrating AI in Traditional Journalism Workflows?
Steering AI integration is like Odysseus’s journey; you face editorial ethics dilemmas, workflow automation hurdles, and data privacy concerns. Technology adoption demands bridging skill gaps, while balancing audience engagement remains essential for seamless adaptation in traditional journalism.
How Do Machine Learning Models Handle Breaking News Scenarios?
You leverage machine learning models for real-time analysis, enabling dynamic reporting during breaking news. These models automatically process data, providing insights quickly, ensuring you’re informed with up-to-the-minute updates, making your reporting more accurate and timely.
What Role Does Machine Learning Play in Combating Fake News?
Imagine a digital watchdog sniffing through data. You train machine learning to automate fake news detection and credibility assessment. It sifts through oceans of information, identifying falsehoods, offering you insightful, data-driven truth amidst the chaos.
How Is Machine Learning Used to Analyze Visual Data in Journalism?
You use machine learning to enhance visual storytelling by employing image recognition algorithms. These algorithms automate the analysis of visual data, providing insightful, data-driven narratives that enrich journalism with precise and reliable content.
Conclusion
In AI journalism, don’t put all your eggs in one basket. Embrace the power of data collection, NLP, sentiment analysis, and automated content generation to enhance storytelling. Use personalization algorithms to tailor news for readers, ensuring engagement and relevance. Always keep ethical considerations front and center to maintain trust. By integrating these techniques, you’ll achieve insightful, data-driven journalism that’s both efficient and impactful, paving the way for the future of news.