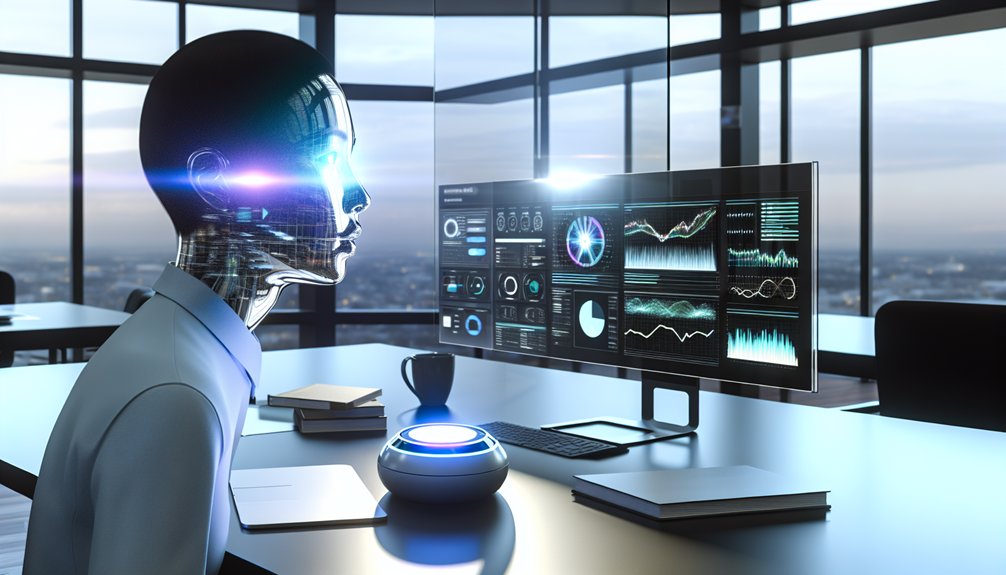
By leveraging AI, you can enhance data analysis with predictive analytics, extracting trends and behaviors from historical datasets. Utilize machine learning for feature selection; employ NLP to interpret text data. With deep learning, refine image recognition capabilities. AI-driven tools enhance data visualization, creating interactive insights. Automate data cleaning to maintain integrity, and integrate AI for scalable data management. AI optimizes cloud storage, boosts system performance, and strengthens data security. Dive deeper to uncover more possibilities.
Leveraging Machine Learning for Predictive Analytics
When you harness machine learning for predictive analytics, you reveal the potential to anticipate future trends and behaviors based on historical data. By implementing feature selection techniques, you can refine your model to focus on the most influential variables, enhancing both efficiency and accuracy. Techniques like recursive feature elimination or LASSO help in identifying these key features. As you construct your models, it’s essential to evaluate their performance using robust model evaluation metrics. Metrics such as accuracy, precision, recall, and F1-score offer insights into the model’s effectiveness and reliability. By understanding these techniques and metrics, you can optimize predictive models, ensuring they not only forecast accurately but also adapt to dynamic data environments, ultimately driving informed decision-making.
Utilizing Natural Language Processing for Text Data
Leveraging the power of Natural Language Processing (NLP) transforms how you analyze and understand text data, allowing machines to interpret and generate human language with remarkable accuracy. By employing text summarization techniques, you can distill vast amounts of information into concise, relevant summaries, enhancing decision-making processes. NLP enables you to quickly grasp the core ideas without wading through extensive documents. Additionally, sentiment analysis applications empower you to gauge public sentiment, providing valuable insights into consumer opinions and market trends. Analyzing social media, reviews, and feedback becomes more efficient, helping you adapt strategies in real-time. These tools not only streamline data analysis but also equip you with a deeper understanding of human language nuances, driving more informed, strategic decisions.
Implementing Deep Learning for Image Recognition
While deep learning technology continues to evolve, its application in image recognition has become increasingly sophisticated and essential. You’ll find that image classification techniques have transformed how computers understand visual data. By leveraging advanced neural network architectures, such as convolutional neural networks (CNNs), you can train models to achieve high accuracy in complex tasks. These networks mimic human visual processing, automatically learning features like edges and textures from raw pixel data. As a practitioner, you should explore various architectures, including ResNet and Inception, each offering unique strengths in handling intricate image datasets. Recognizing the role of hyperparameter tuning is vital for optimizing model performance. The integration of deep learning in image recognition not only enhances data analysis but also pushes the boundaries of AI capabilities.
Enhancing Data Visualization With AI Tools
You can harness AI-driven visualization techniques to uncover patterns and insights from complex datasets with unprecedented clarity. By incorporating interactive data representation, you enable users to engage dynamically with the data, facilitating a more intuitive understanding. This approach not only enhances the interpretability of visual data but also empowers decision-makers to act swiftly and informedly.
AI-Driven Visualization Techniques
Artificial Intelligence transforms how we visualize data, making complex datasets more comprehensible and actionable. By leveraging AI, you can enhance data storytelling through visual storytelling techniques. Utilize 3D visualization and dynamic dashboards to boost user engagement. Design principles are essential; they guide the creation of interactive graphics and augmented reality experiences, making data more immersive. Infographic creation benefits from AI’s ability to apply color theory intelligently, ensuring clarity and appeal. These AI-driven techniques enable you to craft narratives that deeply resonate with your audience, turning raw data into compelling stories. By integrating these advanced tools, you can not only visualize data effectively but also drive insights that support informed decision-making and strategic planning.
Interactive Data Representation
When harnessing AI tools for interactive data representation, you open a new domain of possibilities for engaging with complex information. AI-driven dashboards transform static data into dynamic, immersive experiences. By leveraging dashboard interactivity, you enable users to drill down into datasets, uncovering hidden patterns and insights in real-time. This interactivity fosters a deeper level of user engagement, allowing stakeholders to tailor their exploration to specific queries. AI algorithms can automatically adjust visual elements based on user input, ensuring that the most relevant information is highlighted. This adaptability not only enhances comprehension but also empowers users to make data-driven decisions swiftly. As AI continues to evolve, your ability to interact with data in meaningful ways will become increasingly sophisticated and intuitive.
Improving Data Cleaning and Preprocessing With Automation
Effective data cleaning and preprocessing are essential components of any robust data analysis pipeline, and automation can greatly enhance these processes. By leveraging automated data validation, you can guarantee data integrity and accuracy before analysis begins. This technology checks for missing, duplicate, or inconsistent data entries, allowing you to focus on more strategic analysis tasks. Intelligent outlier detection further refines your dataset by identifying anomalies that could skew results, guaranteeing a cleaner, more reliable dataset.
Incorporating these automated systems not only saves time but also reduces human error, providing a more scalable solution as data volumes grow. By automating these initial steps, you streamline your workflow, allowing you to allocate resources more effectively and concentrate on deriving actionable insights from your data analysis.
Employing AI for Real-Time Data Analysis
Having streamlined the data cleaning and preprocessing phases, you’re now positioned to harness the power of AI for real-time data analysis. This approach empowers you to extract real-time insights and respond promptly to dynamic conditions. Here’s how you can implement it:
- Integrate AI Models: Deploy AI models that ingest continuous data streams, enabling real-time insights without manual intervention.
- Set Up Automated Alerts: Configure AI to trigger automated alerts when key metrics deviate from predefined thresholds, allowing immediate attention to potential issues.
- Optimize Decision-Making: Use AI-driven analytics to enhance decision-making processes by providing actionable insights as events unfold.
Optimizing Data Mining Processes With AI Algorithms
Advanced AI algorithms can greatly streamline data mining processes by automating complex analytical tasks and uncovering patterns that may elude traditional methods. You’ll find that data augmentation plays a significant role in enhancing the quality and quantity of data, enabling more robust model training. By expanding your dataset, you improve predictive accuracy and uncover insights that might otherwise remain hidden. Algorithm optimization is another vital aspect, allowing you to fine-tune AI models for peak performance. This involves adjusting parameters and employing techniques like hyperparameter tuning to maximize efficiency. As a result, your data mining processes become more agile and insightful, empowering you to make informed decisions based on thorough data analysis. Embrace these advancements to propel your data initiatives forward.
Integrating AI in Big Data Management Systems
Building on the optimization of data mining processes through AI algorithms, integrating AI in big data management systems opens new dimensions of data handling capabilities. You’ll see enhanced data integration and system scalability, essential for managing vast datasets. AI-driven metadata management guarantees efficient data retrieval and superior performance optimization. Consider these benefits:
- Data Security: AI enhances your data governance strategies, identifying vulnerabilities and protecting sensitive information.
- Cloud Storage: Implement AI to optimize storage solutions, reducing costs and improving accessibility.
- Infrastructure Management: AI supports analytics frameworks, automating infrastructure decisions to maintain system efficiency.
Frequently Asked Questions
How Can AI Help in Reducing Data Analysis Costs?
You can achieve cost efficiency in data analysis by implementing AI, which automates processes and enhances resource optimization. AI reduces manual labor, accelerates data processing, and minimizes errors, ultimately lowering operational costs and improving analytical accuracy.
What Ethical Considerations Arise When Using AI in Data Analysis?
When using AI in data analysis, you’ll face ethical issues like bias mitigation and maintaining transparency standards. Guarantee your algorithms don’t perpetuate biases while keeping your processes open and understandable to maintain trust and accountability.
How Does AI Handle Data Privacy Concerns in Analysis?
You’ll find AI manages data privacy concerns by incorporating data encryption techniques and adhering to privacy regulations. It guarantees sensitive information remains secure, aligning with compliance standards while processing data efficiently and maintaining user trust.
What Are the Limitations of AI in Data Analysis?
You face limitations in AI data analysis with bias detection, as algorithms can perpetuate existing biases. Interpretability challenges arise when complex models create results that are hard to understand, making it essential to balance accuracy and transparency.
How Does AI Impact the Role of Human Analysts?
AI impacts your role by fostering analyst collaboration and prompting skill evolution. You’ll find yourself integrating AI tools to improve efficiency, while honing strategic thinking and decision-making skills, ensuring your expertise complements AI’s capabilities seamlessly.
Conclusion
In the domain of data analysis, AI acts as the alchemist, transforming raw data into gold. By harnessing machine learning for predictive insights, NLP for text interpretation, and deep learning for image recognition, you unveil new dimensions of understanding. AI tools sharpen your visualization, streamline cleaning, and automate real-time analysis, elevating your data mining to an art. As you weave AI into big data management, it becomes the compass guiding your journey through the data landscape.