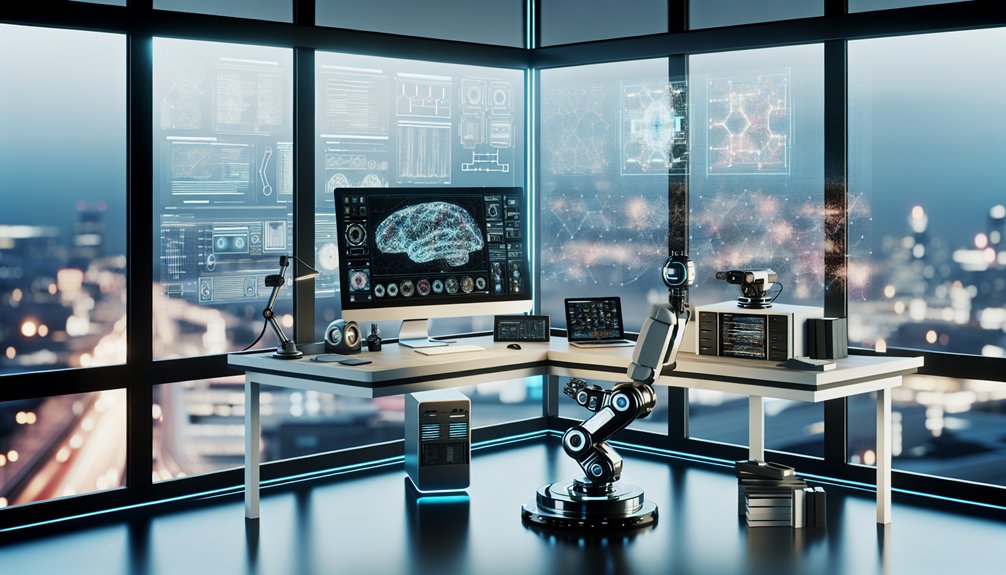
You’re in a world where AI analysis is rapidly advancing. Integration with big data transforms raw input into actionable insights, while explainable AI models improve clarity and trust. Natural Language Processing advances enable more intuitive user interactions. AI’s expansion into edge computing and predictive analytics optimizes real-time decisions. Enhanced cybersecurity tactics protect against sophisticated threats. AI ethics frameworks guarantee responsible deployment. For a deeper understanding, there’s much more to uncover.
Integration of AI With Big Data Analytics
As the digital landscape expands, integrating AI with big data analytics becomes imperative for leveraging complex datasets to drive insightful decisions. You must harness AI-driven automation to streamline data analysis, transforming raw data into actionable insights efficiently. By implementing robust data integration strategies, you can guarantee seamless access to diverse data sources, facilitating a unified view of your information ecosystem. This integration not only enhances your predictive capabilities but also optimizes decision-making processes. With AI-driven automation, you reduce manual intervention, allowing for real-time data processing and swift adaptation to emerging trends. By focusing on innovative data integration strategies, you’re equipped to tackle the challenges of big data, guaranteeing your organization stays ahead in a rapidly evolving technological landscape.
Rise of Explainable AI Models
You’re witnessing a pivotal shift as explainable AI models enhance transparency in complex systems. These models allow you to clearly interpret decision-making processes, fostering trust and accountability. By prioritizing interpretability, you’re setting the stage for AI systems that are not just powerful, but also understandable and reliable.
Enhancing Model Transparency
While AI technologies advance at an unprecedented pace, the demand for transparency within these models grows just as rapidly. You need to understand how model auditing and feature importance play vital roles in enhancing model transparency. By auditing models, you can systematically evaluate their internal workings, identifying potential biases and ensuring compliance with ethical standards. This process not only builds trust but also facilitates accountability.
Feature importance helps you discern which variables markedly impact model predictions, offering a clear view of its decision-making process. By quantifying the contribution of each feature, you gain insights into the model’s logic, ensuring it aligns with expected outcomes. Embracing these strategies allows you to develop AI systems that are not only powerful but also understandable and trustworthy.
Improving Decision Interpretability
In your pursuit of enhancing AI’s decision interpretability, you’ll find that the rise of explainable AI models marks a pivotal shift towards more transparent and accountable systems. These models aim to demystify the decision-making process, offering decision clarity by leveraging robust interpretability frameworks. You’re not just interested in outcomes; you want to understand the “why” behind them. Explainable AI provides insights into complex algorithms, making them comprehensible even to non-experts. By adopting these models, you empower stakeholders with the ability to scrutinize AI decisions, fostering trust and facilitating informed decision-making. As you explore these frameworks, you’ll discover innovative methods like SHAP and LIME, which enhance interpretability by attributing importance to each feature in a model’s decision process.
Advancements in Natural Language Processing
Natural Language Processing (NLP) has undergone remarkable advancements, reshaping how machines understand and interact with human language. You’ll find that semantic understanding now allows systems to grasp context and nuances, improving the accuracy of responses in various applications. This has led to the development of sophisticated conversational agents capable of engaging in more natural and meaningful dialogues. By leveraging deep learning models, these agents can decode complex linguistic structures, enabling them to assist users in tasks ranging from customer service to personal assistants. With the integration of real-time processing and extensive language models, NLP solutions provide more intuitive user experiences. As you explore these developments, you’ll see how they transform machine-human interaction, offering both challenges and opportunities in AI.
Growth of AI in Edge Computing
In the domain of edge computing, AI has markedly improved processing efficiency by minimizing latency and optimizing resource allocation. You can achieve enhanced data privacy by keeping sensitive information local, reducing the need for transmission to central servers. Furthermore, AI’s integration into edge devices empowers real-time decision-making, providing instantaneous insights and actions essential for dynamic environments.
Increased Processing Efficiency
Edge computing represents a significant leap forward in increasing processing efficiency for AI applications. By moving data processing closer to the source, you’re harnessing the power of automated optimization and precise resource allocation. This approach minimizes latency and enhances real-time decision-making. Consider these benefits:
- Reduced Latency: Processing data at the edge reduces the time it takes for information to travel, allowing immediate responses essential for time-sensitive tasks.
- Bandwidth Efficiency: By processing data locally, you’re cutting down on the bandwidth required to transmit data to central servers, optimizing network resources.
- Scalability: Edge computing allows you to scale AI operations seamlessly, as resources can be dynamically allocated based on current demands.
These advancements guarantee your AI applications are not only faster but also more efficient.
Enhanced Data Privacy
As AI continues to expand at the edge, bolstering data privacy becomes imperative. You need to guarantee that sensitive information remains secure, especially as devices handle data locally. Data encryption now plays a pivotal role in safeguarding this information. By encrypting data at the point of creation, you greatly reduce the risk of unauthorized access. Additionally, adhering to stringent privacy regulations guarantees that your AI systems remain compliant and trustworthy. These regulations guide you in implementing robust privacy measures, ensuring data doesn’t get mishandled. With innovative thinking, you’ll integrate advanced encryption algorithms directly into edge devices, creating a seamless and secure data ecosystem. This approach not only protects user data but also builds trust in AI technologies deployed at the edge.
Real-Time Decision Making
With data privacy firmly secured, AI systems at the edge now focus on real-time decision making, driving the growth of edge computing. This shift leverages real-time analytics and dynamic modeling to enable immediate responses closer to data sources. You’ll notice that this empowers faster, more efficient operations.
Consider these advancements:
- Decentralized Processing: AI processes data locally, reducing latency and enhancing real-time decision making.
- Scalable Solutions: Edge computing supports dynamic modeling that adapts to changing data patterns, optimizing performance.
- Improved Resource Allocation: Real-time analytics guarantee resources are used efficiently, minimizing bottlenecks.
These innovations represent a significant step forward in AI’s evolution, offering you unprecedented opportunities for responsive, intelligent systems that adapt seamlessly to evolving conditions. Understanding these trends is vital for harnessing AI’s full potential.
Expansion of AI-Driven Predictive Analytics
You’ve likely observed how AI-driven predictive analytics is transforming industries by enabling more accurate forecasting and decision-making. Through AI model optimization, you can enhance the accuracy and efficiency of predictive models, which are critical in sectors like manufacturing and healthcare. Predictive maintenance, a prime example, leverages these advanced models to anticipate equipment failures before they occur, reducing downtime and costs. This proactive approach integrates vast datasets, allowing you to refine algorithms continually, thereby improving output quality. By adopting innovative techniques in AI model optimization, you’re not just reacting to historical data; you’re shaping future outcomes with precision. In doing so, you’re contributing to a paradigm shift where data-driven insights lead to smarter, more agile operations across various industries.
Use of AI for Enhanced Cybersecurity
Harnessing the power of AI for cybersecurity transforms how you protect digital assets against increasingly sophisticated threats. By leveraging advanced algorithms, you can enhance threat detection and preemptively identify potential attacks. AI’s capability to execute real-time behavioral analysis allows you to discern unusual patterns, ensuring swift responses to anomalies. Here’s how AI enhances your cybersecurity framework:
- Automated Threat Detection: AI systems continuously monitor network traffic, identifying threats that human analysts might overlook.
- Behavioral Analysis: AI models learn from data patterns, enabling you to detect deviations indicative of cyber threats.
- Predictive Insights: AI anticipates future vulnerabilities, allowing you to fortify systems proactively.
Development of AI Ethics and Governance Frameworks
As AI systems become more integrated into daily operations, it’s essential to establish robust ethics and governance frameworks to guide their development and deployment. You need to focus on ethical considerations, guaranteeing AI technologies operate within clear accountability measures. Policy development should involve stakeholder engagement to address diverse perspectives. Implement compliance standards to align with regulatory guidelines and perform regular risk assessments to identify potential issues. Transparency initiatives are critical for fostering trust and understanding. Bias mitigation strategies must be prioritized to prevent unfair outcomes. By crafting effective governance frameworks, you’re not only adhering to current standards but also paving the way for innovative AI solutions that are ethical and equitable. This strategic approach guarantees AI’s responsible and sustainable integration into society.
Frequently Asked Questions
How Is AI Impacting the Job Market and Employment Opportunities?
AI’s reshaping the job market by driving job automation and demanding skill transformation. You’ll find that routine tasks are increasingly automated, freeing you to focus on creative and strategic roles. However, it also means you need to adapt, enhancing skills in AI-related areas. This transformation fosters innovation and requires analytical precision. Embrace these changes by cultivating expertise in emerging technologies, ensuring your relevance in a rapidly evolving landscape.
What Are the Environmental Impacts of Increased AI Usage?
You’re examining how increased AI usage affects the environment. AI resource consumption demands significant energy, often leading to higher carbon emissions. As you explore deeper, consider AI waste management, which involves handling outdated hardware responsibly to reduce e-waste. By innovating in energy efficiency and recycling, you can mitigate these impacts. Your analytical precision in evaluating resource use and waste management will guide sustainable AI practices, balancing technological growth with ecological responsibility.
How Do AI Technologies Influence Consumer Privacy and Data Security?
Imagine an app that tracks your fitness without your knowledge. AI technologies can greatly affect consumer privacy and data security. When data ethics are overlooked, privacy breaches occur, undermining trust. To navigate this, you must guarantee strong security protocols, adhere to privacy regulations, and prioritize consumer consent. Innovative solutions require analytical precision to balance technological benefits with ethical considerations, safeguarding users while leveraging AI’s potential. Understanding these dynamics is essential for responsible AI deployment.
What Are the Challenges in Ai-Human Collaboration in the Workplace?
You’re traversing a complex landscape when integrating AI into the workplace. Communication barriers often arise, as AI systems may not interpret human nuances accurately. Trust issues can develop if AI decisions seem opaque or unpredictable. To overcome these challenges, foster transparency and encourage cross-disciplinary collaboration. Equip yourself with technical expertise and analytical precision, enabling you to bridge gaps and harness AI’s potential for innovative solutions and efficient workflows.
How Is AI Changing the Landscape of Education and Learning?
How do you see AI revolutionizing education’s future? With personalized learning, AI tailors curriculum to individual student needs, ensuring effective, engaging experiences. Virtual classrooms break geographical barriers, democratizing access to quality education. You’re witnessing AI analyze vast data sets, identifying gaps and strengths, and suggesting improvements. This innovative approach fosters a dynamic learning environment, encouraging analytical thinking and adaptability, essential skills in an ever-evolving world. AI’s impact on education is profound and transformative.
Conclusion
You’re standing at the frontier of AI analysis, where the fusion of AI with big data analytics acts as a catalyst for groundbreaking innovations. As explainable AI models rise, they unravel complexity like peeling back the layers of an onion. Advancements in natural language processing and growth in edge computing revolutionize interactions and efficiency. With predictive analytics, cybersecurity enhancements, and ethical frameworks, you’re equipped to harness AI’s transformative power, driving precision and innovation in today’s digital landscape.