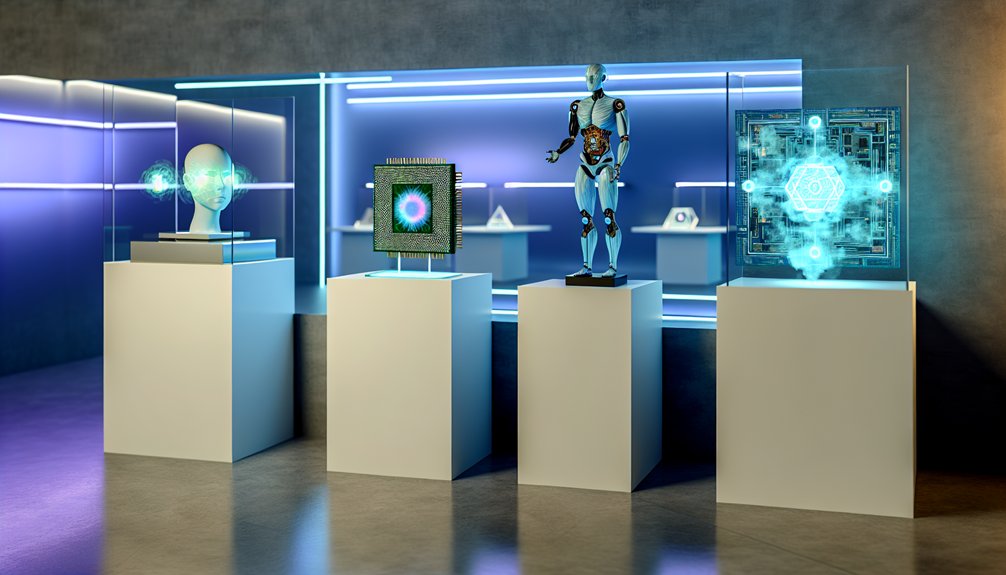
The top three recent innovations in AI and machine learning are revolutionizing the field. Transformer-based language models, with their self-attention mechanisms, enhance language understanding by weighing word importance contextually. Self-supervised learning techniques utilize vast unlabeled data, improving model efficiency and feature extraction. Reinforcement learning advancements optimize decision-making and exploration strategies in diverse environments. These developments enable robust, scalable solutions, expanding AI’s practical applications. Interested in uncovering more about how these innovations are shaping AI’s future?
Transformer-Based Language Models
When exploring the landscape of artificial intelligence, transformer-based language models stand out as a pivotal innovation. You’ll find their transformer architecture fundamentally alters language understanding, enabling complex computations on vast textual data. This architecture leverages self-attention mechanisms, allowing the model to weigh the importance of different words in a sentence contextually. It doesn’t just process sequences serially but considers the relationships between all words simultaneously. This approach enhances language understanding, capturing subtleties and nuances more effectively than previous models. With its ability to handle large-scale data and parallelize tasks, transformer architecture has revolutionized natural language processing applications. It’s not just about processing language data efficiently; it’s about transforming how machines interpret and generate human-like text, facilitating advanced AI solutions.
Self-Supervised Learning Techniques
Transformer-based language models have redefined language processing, and now self-supervised learning techniques are pushing boundaries in AI model training. These techniques leverage vast amounts of unlabeled data, greatly enhancing data efficiency and broadening self-supervised applications. Here’s how self-supervised learning is transforming AI:
- Data Utilization: By harnessing unlabeled datasets, models learn more effectively without requiring extensive labeled data, reducing costs and time.
- Feature Extraction: Self-supervised techniques excel at extracting features, improving model accuracy and decision-making.
- Pre-training Models: This method pre-trains models on generic tasks, enabling quicker adaptation to specific tasks with minimal additional data.
- Improved Adaptability: Models become more adaptable to new tasks by learning from diverse data, enhancing their robustness and generalization capabilities.
These advancements drive AI’s evolution, making models more efficient and effective.
Reinforcement Learning Advancements
Reinforcement learning (RL) has greatly evolved, revolutionizing the way systems learn and adapt through interaction with their environment. You’ll find policy optimization at the heart of modern RL, enhancing decision-making by refining the policy directly. This leads to more efficient learning processes. Exploration strategies, another key component, guarantee that RL agents discover ideal paths in diverse environments, balancing the trade-off between exploration and exploitation.
Innovation | Impact | Emotion |
---|---|---|
Policy Optimization | Enhanced Efficiency | Amazement |
Exploration | Adaptive Learning | Curiosity |
Robust Algorithms | Stability in Results | Confidence |
Scalable Solutions | Broader Applications | Excitement |
Real-world Testing | Practical Relevance | Satisfaction |
These advancements are vital for applications ranging from autonomous systems to strategic game playing, offering robustness and scalability that weren’t possible before.
Frequently Asked Questions
How Does AI Impact Job Markets and Employment Rates?
AI’s impact on job markets is significant. You see job displacement as machines take over repetitive tasks. This shift creates a noticeable skills gap; workers need to upskill or reskill to stay relevant. Data shows automation affects industries differently, with manufacturing experiencing higher displacement rates. However, AI also generates jobs in tech, requiring analytical skills. Understanding these dynamics helps you navigate the evolving employment landscape effectively, ensuring you’re prepared for future challenges.
What Ethical Concerns Arise With AI Advancements?
You’re examining ethical concerns in AI advancements, focusing on bias mitigation and accountability frameworks. Bias in AI can skew data-driven decisions, so you must implement robust bias mitigation strategies. Accountability frameworks guarantee that AI systems operate ethically, holding developers responsible for their creations’ impacts. By analyzing data patterns and applying technical solutions, you’ll navigate the complexities of AI ethics, guaranteeing transparent and fair systems that align with societal values.
How Can AI Contribute to Climate Change Solutions?
You might think AI is just tech hype, but it plays an essential role in combating climate change. By enhancing carbon tracking, AI gives you precise data on emissions, enabling better decision-making. It optimizes renewable energy sources, predicting demand and adjusting supply efficiently, reducing waste. AI’s data-driven insights help you understand complex climate patterns, supporting strategies that mitigate environmental impact. Embrace AI’s potential for a sustainable future.
What Are Current AI Applications in Healthcare?
You’re seeing AI transform healthcare with its capability to enhance diagnostic accuracy and tailor personalized medicine. By analyzing vast datasets, AI algorithms identify patterns and predict disease outcomes with unprecedented precision. This data-driven approach aids in developing bespoke treatment plans, ensuring patients receive the most effective therapies. AI’s analytical power reduces human error, accelerates diagnosis, and optimizes resource allocation, ultimately improving patient care and health outcomes considerably.
How Is AI Improving Cybersecurity Measures?
You might wonder how AI is enhancing cybersecurity measures. AI’s role in threat detection is pivotal, analyzing vast datasets to identify anomalies and predict potential breaches. It leverages machine learning algorithms to continuously learn and adapt, improving accuracy over time. Additionally, AI strengthens data encryption by developing more robust algorithms, ensuring sensitive information remains secure. By automating these processes, AI not only increases efficiency but also reduces human error in cybersecurity protocols.
Conclusion
In your exploration of recent AI innovations, you’ve seen the transformative power of transformer-based language models, self-supervised learning, and reinforcement learning. One striking detail is that GPT-3, a transformer model, boasts 175 billion parameters, showcasing an unprecedented leap in natural language processing capabilities. These advancements aren’t just incremental; they’re reshaping AI’s landscape, enabling machines to learn more efficiently and effectively. As these technologies evolve, you’re witnessing a pivotal moment in AI’s development, promising even greater breakthroughs ahead.