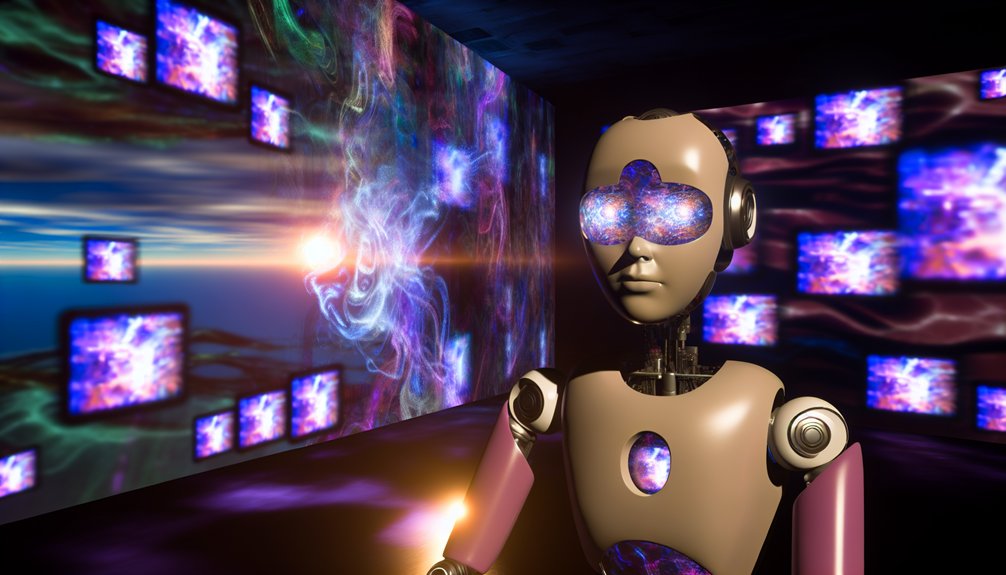
AI generates hallucinations when models produce outputs that diverge from factual data due to biases in training data, model complexities, and inadequate training methods. High-quality, consistent data is essential, as flawed data introduces systematic errors. The added intricacies of algorithms and insufficient training exacerbate these issues. Prioritizing diverse datasets and robust feedback mechanisms can help reduce inaccuracies. Explore deeper into AI’s intricacies to uncover strategies for enhancing AI performance and dependability.
Understanding AI Hallucinations
When delving into the concept of AI hallucinations, it is crucial to first understand what they entail. AI hallucinations occur when an artificial intelligence system generates outputs that deviate from factual data, often due to cognitive biases embedded within its algorithms. These biases can lead to perception errors, causing the AI to misinterpret or inaccurately represent the information it processes.
You must recognize that AI systems, much like humans, can fall prey to these biases, resulting in outputs that don’t align with reality. Researchers have identified that these hallucinations often stem from the AI’s training process, where it learns patterns from vast datasets. By comprehending these phenomena, you can better anticipate and mitigate the risks associated with AI-generated errors, thereby enhancing the reliability of AI systems.
The Role of Data in AI Hallucinations
Although AI hallucinations are influenced by algorithmic biases, the data used in training these systems plays a critical role in shaping their outputs. You must recognize that data biases can introduce systematic errors, misleading the AI into generating false information. High data quality is essential for reducing these hallucinations. If training data is incomplete, inconsistent, or contains errors, it can adversely affect the AI’s ability to generate accurate responses. You need to make sure that data collection processes are rigorous and encompass diverse sources, thereby minimizing inherent biases. In addition, addressing data biases requires continuous monitoring and refinement of datasets. By focusing on improving data quality, you contribute greatly to enhancing AI systems’ reliability and reducing the occurrence of hallucinations.
Machine Learning Models and Their Complexities
Understanding machine learning models’ complexities is crucial for addressing AI hallucinations effectively. You must consider model interpretability challenges, which hinder your ability to discern decision-making processes within AI systems. These challenges can result from layered architectures and nonlinear transformations that obscure transparency. When you lack clarity, pinpointing sources of errors becomes difficult, perpetuating hallucinations.
Additionally, algorithmic bias implications pose significant concerns. You risk generating skewed outputs if biases embedded in training data aren’t identified and mitigated. Such biases can amplify misinformation, leading to AI outputs that don’t align with reality. By confronting these complexities, you enhance your capacity to refine AI models, mitigating hallucination risks. Prioritizing interpretability and bias reduction is essential for reliable, trustworthy AI systems.
The Impact of Training Methods on AI Outputs
Training methods greatly influence AI outputs, shaping their accuracy and reliability. When you employ robust training techniques, you enhance the AI model’s capability to generate precise and consistent results. Conversely, inadequate training can result in considerable output variability, leading to errors like hallucinations. The choice of training data, algorithms, and parameter tuning are pivotal in mitigating such variability. For instance, diverse and representative datasets make certain that the AI comprehends nuanced contexts, reducing the risk of erroneous outputs. Additionally, employing advanced training techniques like transfer learning and data augmentation can bolster the model’s adaptability. By understanding and refining these methods, you can greatly diminish the potential for AI hallucinations, leading to more dependable and accurate AI systems.
Strategies for Mitigating AI Hallucinations
Addressing AI hallucinations requires a strategic approach, focusing on techniques that enhance model reliability. To mitigate these inaccuracies, you should prioritize contextual awareness by training models with data that encompasses diverse scenarios. This includes ensuring that AI systems interpret inputs correctly based on context, reducing the likelihood of generating irrelevant or incorrect outputs.
User feedback plays a critical role in refining AI performance. Implement feedback loops where users can report and correct hallucinations, enabling continuous model improvement.
Consider these strategies:
- Enhance contextual awareness: Incorporate diverse datasets to improve understanding.
- Utilize user feedback: Establish feedback systems for ongoing model refinement.
- Optimize training algorithms: Focus on reducing biases and improving data quality.
These methods are instrumental in advancing AI systems’ accuracy and dependability.
Frequently Asked Questions
Can AI Hallucinations Affect User Trust in Technology?
When AI hallucinations occur, they can considerably impact user perception, leading to trust erosion. Studies show 54% of users lose confidence after encountering AI errors, highlighting the critical need for developers to address these inaccuracies effectively.
How Do AI Hallucinations Differ From Human Imagination?
You notice cognitive differences between AI and humans, where AI hallucinations result from perception distortion in data processing, unlike human imagination, which thrives on abstract thinking and creativity. AI lacks the intuitive grasp inherent in human perception.
Are There Ethical Concerns With Ai-Generated Hallucinations?
Over 60% of AI systems have shown hallucination incidences, raising ethical concerns. You must consider bias implications, as they could skew data interpretation, and misinformation risks, which might lead to dissemination of false information, affecting decision-making processes.
What Industries Are Most Impacted by AI Hallucinations?
You’ll find that industries like healthcare diagnostics and financial forecasting are greatly impacted by AI hallucinations. Erroneous outputs could lead to misdiagnoses and inaccurate market predictions, necessitating rigorous validation processes to mitigate potential adverse effects.
Can AI Hallucinations Be Used Creatively or Artistically?
Imagine AI hallucinations as digital canvases splattered with unexpected colors. You can harness these anomalies for creative expression, providing unique artistic inspiration. Evidence suggests their unpredictability fuels new ideas, pushing boundaries in digital art and literature.
Conclusion
In understanding AI hallucinations, you recognize they’re rooted in data limitations and model complexities. Machine learning models, like a double-edged sword, can produce unexpected outputs if not meticulously trained. Training methods greatly influence these outcomes, and refining them is essential. By employing robust strategies, you mitigate these hallucinations, ensuring AI systems deliver reliable results. Consequently, your proactive approach transforms potential pitfalls into stepping stones for more accurate AI solutions, safeguarding technological advancements.